About Machine Learning
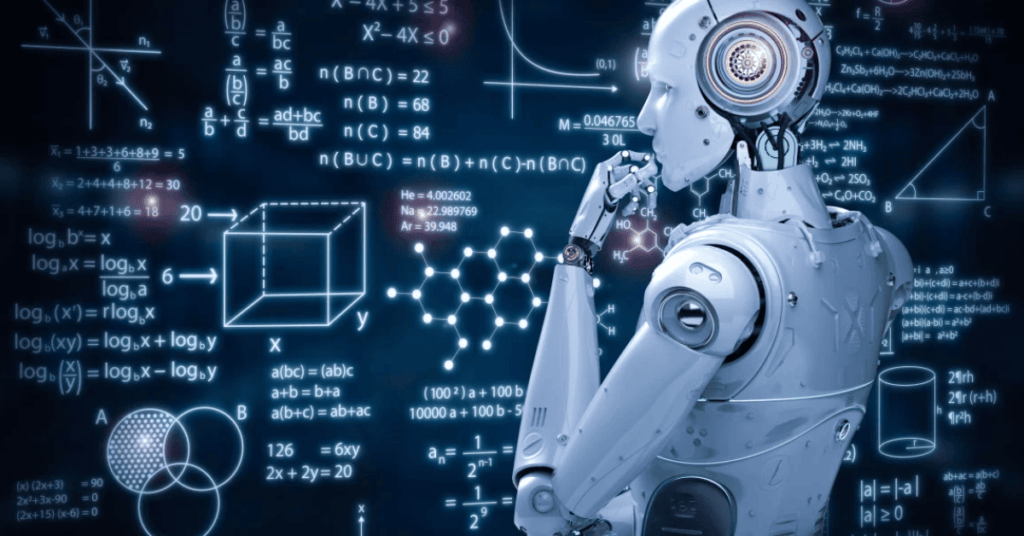
Machine Learning (ML) continues to grow in importance, bringing innovations that impact everyday life and industries worldwide. As we enter 2024 , several trends stand out in reshaping how ML works and what it can do. Here ’s a straightforward look at the top seven trends you should know about.
Multimodal Machine Learning: Combining Different Data Sources
Multimodal ML lets systems process and interpret multiple types of information, such as text, images. and sounds, all at once. This technology is already making virtual assistants smarter and more interactive. Imagine asking an AI to analyze a photo while explaining what it sees—multimodal ML makes that possible. It ’s being used in areas like medical imaging, education, and customer service to deliver richer results.
Explainable AI (XAI): Making AI More Transparent
One major issue with ML models is that they can feel like “black boxes.” They give answers, but it ’s often unclear how they reached those conclusions. Explainable AI solves this by showing why an algorithm made a decision. This is especially useful in fields like healthcare and finance, where understanding AI’s reasoning is critical to ensuring fairness and building trust.
Reinforcement Learning: Smarter Systems Through Rewards
Reinforcement learning works by rewarding correct actions and discouraging wrong ones, helping AI systems learn over time It ’s like training a pet—you give it treats when it behaves well. This technique is being used in robotics, gaming, and autonomous vehicles to create smarter and more adaptable systems.
Low-Code and No-Code ML: Making AI Accessible
Not everyone is a programmer, but with low-code and no-code platforms, you don’t need to be. These tools let businesses and individuals build AI models using simple interfaces without heavy coding. This trend is opening doors for small businesses to use ML for tasks like fraud detection, hiring and improving customer experiences.
Quantum Machine Learning: Faster Problem-Solving
Quantum computing is merging with ML to solve problems that regular computers can’t handle efficiently. For example, quantum ML can be used to speed up drug discovery, optimize supply chains , and even improve cryptography. While it ’s still an emerging field, its potential is enormous.
Real-Time Personalization: Tailored Experiences in the Moment
Real-time ML adapts instantly to user behavior. For example, online shopping sites can update recommendations while you browse. or streaming services can suggest content based on your viewing habits. This trend makes customer experiences more personalized and engaging, which helps businesses retain users.
MLOps: Managing ML Models at Scale
MLOps, short for Machine Learning Operations, focuses on managing and maintaining ML systems efficiently. It ensures that models perform well over time by addressing issues like outdated predictions and improving scalability . This is becoming essential as more companies rely on ML systems for daily operations.
Future of Machine Learning
Machine Learning in 2024 isn’t just about new technologies—it ’s about making AI more transparent, accessible, and effective. Whether it’s smarter systems, faster problem-solving , or easier tools for beginners, these trends are shaping the future of AI in meaningful ways.
Frequently Asked Questions(FAQs)
What is Multimodal Machine Learning and how is it used?
Multimodal Machine Learning (ML) combines multiple types of data—like text, images, and audio—into a single system to provide more comprehensive insights. This technology is used in various industries, such as healthcare (for medical imaging and diagnostics), entertainment (for enhancing virtual assistants), and customer service (for chatbots that process both text and voice inputs). By integrating different data sources, multimodal systems can generate richer, more accurate responses.
Why is Explainable AI (XAI) important?
Explainable AI (XAI) is crucial because it helps make AI decision-making more transparent. Traditional AI models, especially deep learning networks, can operate as “black boxes,” meaning they provide outputs without explaining how they reached those conclusions. In industries like healthcare and finance, understanding AI’s reasoning is essential to ensure fairness, trust, and ethical use. XAI is helping to address these concerns by making AI models more interpretable.
How does Low-Code and No-Code Machine Learning make AI accessible?
Low-code and no-code platforms allow people without technical backgrounds to create machine learning models. These platforms provide simple, visual interfaces to build AI applications, making ML accessible to a broader audience, including business professionals. These tools are widely used in tasks like customer analysis, fraud detection, and even recruitment, where businesses can implement AI solutions quickly without needing deep coding knowledge.
What is Quantum Machine Learning and why is it significant?
Quantum Machine Learning (Quantum ML) combines the power of quantum computing with machine learning to solve complex problems faster than traditional computers. Quantum ML is expected to revolutionize industries like drug discovery, financial modeling, and optimization by processing large datasets at unprecedented speeds. While still in its early stages, its potential to transform fields requiring high computational power is immense.