Large Language Models
Large Language Models (LLMs) have become a cornerstone of modern artificial intelligence, transforming how we interact with technology and driving innovations across various sectors. These models, designed to understand and generate human-like text, are playing an increasingly important role in fields like healthcare, customer service, and business operations. As we move into 2024, several trends related to LLMs are shaping their evolution and influence. Let’s explore some of the key trends that are making waves in the AI world.
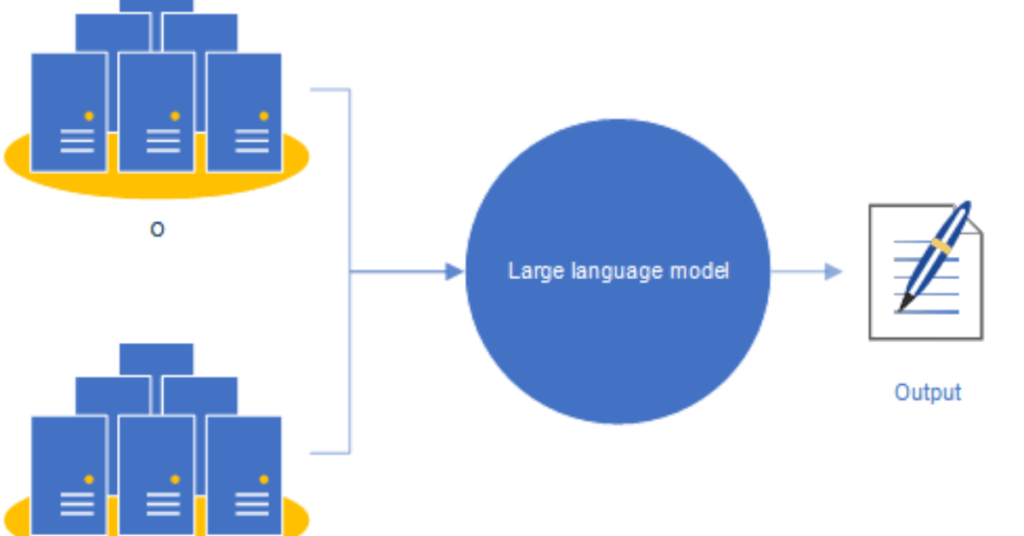
Neural Machine Translation (NMT): Breaking Language Barriers
One of the most impactful uses of LLMs is Neural Machine Translation (NMT) . NMT relies on neural networks to translate text between languages more naturally and accurately than traditional translation systems. Unlike older methods, NMT is context-aware, which makes it ideal for translating complex or nuanced text. This technology is particularly valuable for businesses that need to communicate across different languages in real time, breaking down barriers and expanding global reach. As industries continue to grow internationally, the demand for robust translation tools powered by LLMs is expected to rise.
Voice Intelligence: Enhancing Communication Through AI
Voice Intelligence is another trend that is gaining traction. This technology allows LLMs to process and understand spoken language, extracting meaningful insights from voice data. As speech recognition becomes more accurate, applications for voice-driven AI are expanding in areas such as customer service, virtual assistants, and transcription services. By improving the way machines understand and interact with spoken language, voice intelligence is making communication more seamless and accessible. For example, in customer support t, AI can now handle voice-based queries, delivering faster responses and reducing the need for human agents.
Transfer Learning : Faster AI Training
Transfer Learning is a method that enables LLMs to apply knowledge from one task or domain to another, reducing the need for extensive retraining. This is especially useful for businesses looking to quickly adapt AI models to new areas. For example, if an LLM is trained to understand general text, it can transfer that knowledge to a specialized field like medicine or law with minimal additional training. This method not only accelerates the development of LLMs but also reduces the computational resources needed to train them. It’s a significant development in AI because it makes advanced models more accessible and efficient.
Machine Learning as a Service (MLaaS) : Democratizing AI
Machine Learning as a Service (MLaaS) refers to cloud-based platforms that provide machine learning tools without requiring companies to invest in complex infrastructure. This trend is particularly important for small and medium-sized businesses that want to adopt AI but lack the resources to build their own models. With MLaaS, companies can use powerful LLMs for tasks such as content generation, predictive analytics, and customer insights. This trend is making AI more accessible and allowing businesses of all sizes to harness the power of LLMs.
Automated Speech Recognition (ASR) : A Game Changer for Communication
Automated Speech Recognition (ASR) is the technology behind converting spoken language into written text. ASR is helping businesses improve customer service, transcription services, and virtual assistant functionality. By enabling real-time transcription, ASR is also improving accessibility for people with hearing impairments. In industries like healthcare, ASR is being used to transcribe doctor-patient conversations, ensuring accurate and efficient medical records. This technology is integral to the growing trend of voice-driven AI applications.
Large-Scale Pretraining : Boosting Model Accuracy
Large-scale pretraining refers to training LLMs on massive datasets, allowing them to learn more about language and its nuances. The more data a model is trained on, the better it can understand complex language patterns and generate more accurate responses. This trend is crucial for improving the performance of LLMs in real-world applications, such as customer support, content generation, and data analysis. With access to large amounts of text, LLMs become better at handling various tasks, making them more effective across industries.
Zero-shot and Few-shot Learning : AI Learning with Less Data
Zero-shot and few-shot learning are techniques that allow LLMs to perform tasks with little or no prior training data. In zero-shot learning, the model is able to make predictions or solve problems without seeing any examples, while few-shot learning requires only a few examples. These methods are particularly valuable in situations where annotated data is limited or hard to acquire. For businesses, this means they can apply LLMs to new tasks without having to spend a lot of time and resources on training data. This flexibility is transforming how AI can be applied across industries, even in areas where data is scarce.
Future of Large Language Models
As technology continues to evolve, the impact of Large Language Models will only grow stronger. From breaking language barriers with Neural Machine Translation to enhancing communication through voice intelligence, LLMs are reshaping industries and creating new opportunities. The integration of advanced techniques like transfer learning, large-scale pretraining, and few-shot learning is making these models more efficient, flexible, and accessible. With Machine Learning as a Service and Automated Speech Recognition opening up new possibilities, businesses of all sizes can benefit from these advancements.
The future of LLMs is exciting, and as they continue to evolve, they will play an even more integral role in transforming the way we work, communicate, and innovate. Whether it’s improving customer service, creating more personalized experiences or automating tasks, LLMs are set to revolutionize the world of AI in 2024 and beyond .
Frequently Asked Questions(FAQs)
What are Large Language Models (LLMs)?
Large Language Models (LLMs) are AI models designed to understand and generate human-like text based on vast amounts of data. These models can process complex language patterns, perform tasks like translation, content generation, and even answer questions. LLMs are trained on large datasets, making them powerful tools for a variety of applications.
How does Neural Machine Translation (NMT) work in LLMs?
Neural Machine Translation (NMT) uses neural networks to translate text between different languages. LLMs trained on NMT can provide more accurate translations by understanding context, nuances, and meaning, rather than just literal translations. This technology helps businesses overcome language barriers, improving global communication and accessibility.
What is Transfer Learning and why is it important for LLMs?
Transfer Learning refers to the practice of applying knowledge learned from one task or domain to another. This makes training LLMs more efficient by enabling them to leverage existing knowledge and adapt to new areas with minimal additional data. It speeds up the training process and allows LLMs to be applied to specialized fields with fewer resources.
What is the future of Large Language Models?
The future of LLMs is bright, with ongoing advancements that will make these models more powerful and accessible. As LLMs continue to scale, they will drive innovation across a variety of fields, enhancing communication, improving customer service, and automating complex tasks. With advances in transfer learning, zero-shot learning, and large-scale pretraining, LLMs will become even more adaptable and impactful in the years to come.
Related Posts
- 2-in-1 Laptops: Tablets and Laptops in One
- 5G Technology: What It Is, It Works and Impact on Our Lives
- 8K Gaming on a Laptop: Is It Worth It?
- A Simple Guide to Remote Work: Work from Anywhere, Anytime
- A Visit to the Museum of Jurassic Technology
- Affordable AI Solutions for Small Businesses